Deep Global Registration
Abstract
We present Deep Global Registration, a differentiable framework for pairwise registration of real-world 3D scans. Deep global registration is based on three modules: a 6-dimensional convolutional network for correspondence confidence prediction, a differentiable Weighted Procrustes algorithm for closed-form pose estimation, and a robust gradient-based SE(3) optimizer for pose refinement. Experiments demonstrate that our approach outperforms stateof-the-art methods, both learning-based and classical, on real-world data.
Paper
Oral Presentation
1-min Video
Quick Pipeline Video
Supplementary Materials
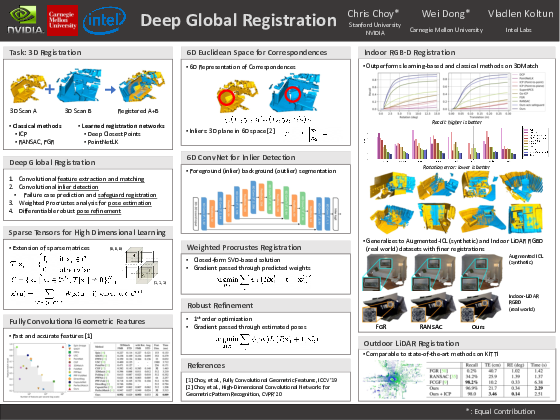
- KITTI registration visualizataion
Registration Results
- All registration results of every 100th frames of the 3DMatch benchmark
Bibtex
@inproceedings{choy2020deep,
title={Deep Global Registration},
author={Choy, Christopher and Dong, Wei and Koltun, Vladlen},
booktitle={Proceedings of the IEEE Conference on Computer Vision and Pattern Recognition},
year={2020}
}
Leave a Comment